An intricate balance of scientific innovation, regulatory rigor, and heavy financial investment has long defined the life science and pharmaceuticals industry. From small molecule drugs to biologics and advanced gene therapies, the journey from initial concept to market can stretch over a decade and cost billions of dollars. This intricate process, commonly called the drug development pipeline, traditionally involves target identification, lead compound discovery, preclinical studies, and clinical trials, culminating in regulatory approval.
However, recent advances in Artificial Intelligence (AI) and Machine Learning (ML)—including the emergence of Large Language Models (LLMs)—are revolutionizing every stage of this pipeline. By leveraging AI/ML algorithms for tasks such as molecular screening, protein structure prediction, toxicity assessment, and even clinical trial optimization, pharmaceutical companies can significantly reduce the time and cost of bringing a new drug to market.
In this article, we will explore how AI/ML technologies are shaping the drug discovery landscape and speeding up the drug development process while offering substantial cost savings and enhancing the competitive edge of life science organizations.
The Traditional Drug Discovery Challenge
Before diving into the specifics of AI/ML applications, it’s crucial to understand the traditional drug discovery challenges:
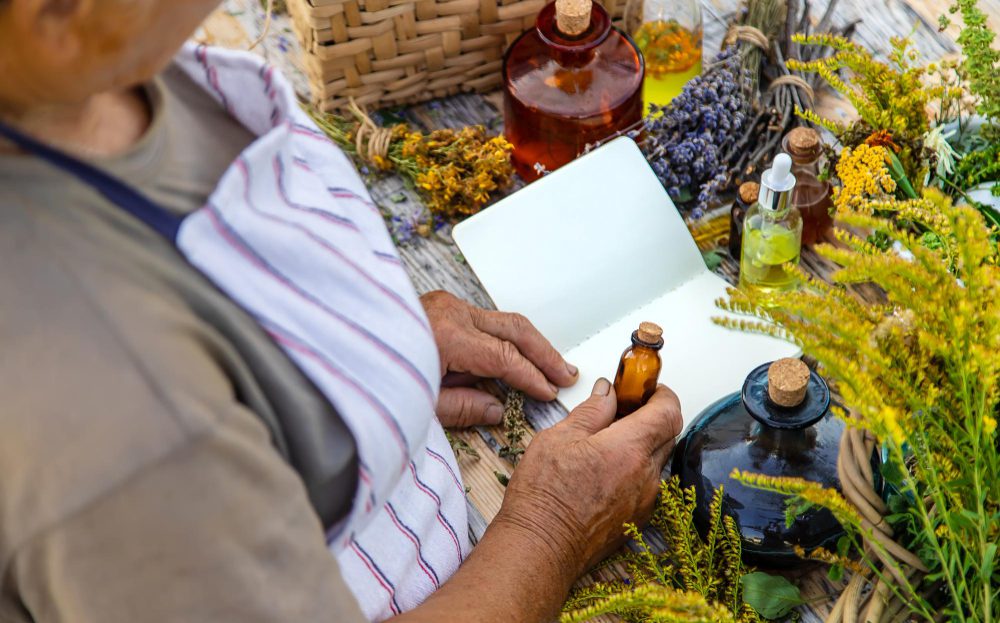
- High Costs: Estimates suggest that bringing a single new medication to market can cost upwards of $2.6 billion. This includes discovery, clinical trials, and post-market surveillance costs.
- Long Timelines: The journey from initial research to FDA approval can span 10 to 15 years. Every stage—discovery, preclinical testing, clinical trials, and regulatory review—is lengthy and resource-intensive.
- High Failure Rates: The success rate from phase I clinical trials to final approval is under 10%, often leading to huge sunk costs and wasted time. Many compounds fail due to safety or efficacy issues discovered late in development.
- Data Overload: The volume of scientific literature and data grows exponentially each year, making it challenging for researchers to stay updated, let alone harness all relevant information for drug discovery.
By harnessing AI/ML and LLM technologies, pharmaceutical companies and life science organizations have started to address these challenges head-on. Let’s examine how.
How AI/ML Is Transforming Drug Discovery
Target Identification and Validation:
One of the earliest and most critical steps in drug development is identifying the biological target, often a protein or a gene, that plays a key role in a disease. AI/ML can rapidly screen through genomic data, proteomic data, and scientific literature to pinpoint the most promising targets for further investigation.
Machine Learning for Biomarker Discovery: Algorithms can detect patterns in large patient datasets to identify biomarkers that correlate with disease progression or drug response. This significantly refines target selection.
LLM-Assisted Literature Review: Large Language Models can parse thousands of scientific publications in minutes, summarizing and highlighting the most relevant studies for specific targets or diseases, accelerating researchers’ ability to make data-driven decisions.
Business Impact: By getting the target identification process right from the outset, pharmaceutical companies can reduce late-stage failures, saving millions of dollars and years of research time.
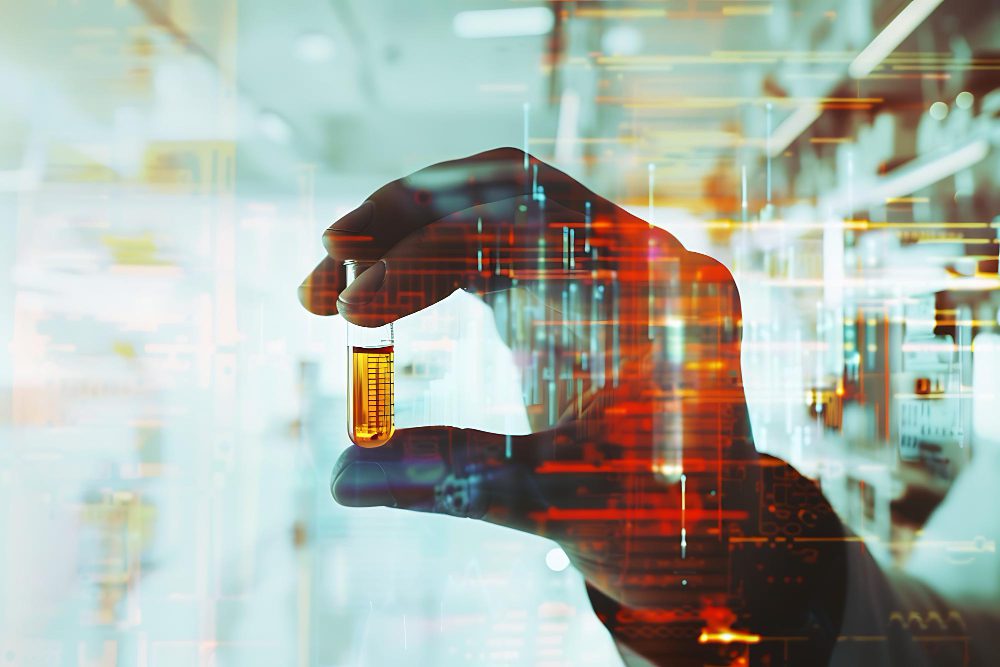
Lead Compound Discovery
Once a biological target is identified, the next step is to find a lead compound that interacts effectively with it. Traditionally, researchers relied on high-throughput screening (HTS) to test thousands or millions of compounds against a target, which is both expensive and time-intensive.
- In Silico Screening: AI-powered in silico models can predict how a new chemical entity might bind to a target, saving researchers from physically testing every candidate. By focusing on those most likely to succeed, labs can cut down on wet lab experiments dramatically.
- Generative Models for Drug Design: Deep learning algorithms can now generate novel molecular structures tailored to a specific target. By considering physicochemical properties, absorption, distribution, metabolism, excretion (ADME), and toxicity, AI can design compounds that have a higher likelihood of success.
- LLM-Powered Databases: Large Language Models can interface with chemical databases, patent repositories, and published literature to identify existing compounds or analogs that show promise, reducing the time spent on redundant research.
Business Impact: Significantly lowers the cost of initial compound screening and accelerates the path to identifying a strong lead candidate—one of the most time-consuming phases of drug discovery.
Optimization and Validation
After discovering a promising lead, extensive optimization is required to ensure it has the right efficacy, selectivity, and safety profile. This optimization process traditionally involves multiple rounds of chemical synthesis and testing, again inflating costs and timelines.
- Predictive Modeling: ML algorithms can predict how small modifications to a chemical structure will affect its binding affinity, solubility, and toxicity. This guides scientists in making data-driven modifications, rather than relying on trial-and-error.
- Toxicity Screening: Early-stage toxicity screening is crucial to avoid expensive failures in later clinical trials. AI-based toxicity prediction models analyze chemical structures and compare them against known toxic compounds, flagging high-risk candidates.
- Automation and Robotics: When integrated with AI insights, robotics can automate repetitive lab processes, such as compound synthesis and assay tests, increasing throughput while reducing human error.
Business Impact: More efficient optimization translates to a higher success rate in preclinical development, drastically reducing the risk of late-stage failures and saving both time and capital.
Pre-clinical and Clinical Trial Streamlining
Even after a compound is optimized in the lab, the real test begins in preclinical studies and clinical trials. These stages ensure safety and efficacy in living systems, and they represent a significant portion of the overall development timeline.
- Animal Model Analysis: ML algorithms can predict which animal models will provide the most relevant data for a particular disease, ensuring more translatable results to human trials.
- Patient Recruitment: AI can sift through electronic medical records (EMRs) and patient databases to identify suitable clinical trial participants based on genetic, demographic, and medical history criteria. This drastically cuts recruitment time, often the biggest bottleneck in clinical research.
- Adaptive Trial Design: Machine learning can drive adaptive clinical trials, where data from ongoing trials are analyzed in real-time to adjust dosing or stratify patients into subgroups more likely to respond positively. This reduces the overall number of subjects needed and speeds up conclusive results.
- Remote Monitoring and Digital Endpoints: AI-enabled wearables and mobile applications can continuously monitor patient vitals, providing real-time data. This not only offers a more accurate view of patient responses but also streamlines trial logistics, enabling decentralized or hybrid trial models.
Business Impact: Streamlining preclinical and clinical stages with AI can shorten the overall development time, reduce patient dropout rates, and optimize resource allocation. Ultimately, this can lead to millions of dollars in savings for life science organizations.
Regulatory Compliance and Post-Market Surveillance
Following successful clinical trials, regulatory approval is the next major milestone. Even post-approval, pharmaceutical companies must keep a close eye on pharmacovigilance and post-market surveillance to monitor adverse events and real-world effectiveness.
- Automated Documentation: LLMs can generate and analyze regulatory submissions, reducing the burden on compliance teams. By automating the creation of Common Technical Documents (CTDs) and other forms, companies save significant administrative overhead.
- Safety Signal Detection: AI can comb through patient reports, medical records, and social media channels to detect early safety signals and adverse events. Quick identification helps manage risks proactively and maintain compliance.
- Real-World Evidence (RWE): Machine learning can analyze electronic health records, insurance claims, and patient-generated data to gauge the long-term efficacy and safety of a drug. This helps in refining labeling and usage guidelines, ensuring ongoing regulatory compliance.
Business Impact: Managing compliance efficiently can prevent costly recalls, litigation, and reputation damage, ensuring long-term profitability and sustainability.
The Role of Large Language Models (LLMs) in Drug Discovery
While the broader AI/ML field focuses on numerical and image-based data analytics, the rise of Large Language Models (LLMs)—like GPT-based architectures—adds a powerful new dimension:
- Literature Summarization: Research teams grapple with huge volumes of scientific papers. LLMs can summarize entire fields of study within minutes, pinpointing crucial findings, thus reducing the time researchers spend sifting through data manually.
- Patent Analysis: Patent searches can be complex and time-intensive. By training an LLM on patent databases, organizations gain quick insights into freedom-to-operate, potential licensing opportunities, and competitive intelligence.
- Hypothesis Generation: LLMs excel at synthesizing information from various sources. They can propose new hypotheses or research directions that may have been overlooked by humans, driving innovation in drug discovery.
- Accelerating Team Collaboration: By using LLMs as interactive knowledge bases, multidisciplinary teams (biologists, chemists, computational scientists) can communicate more effectively, bridging language gaps and fostering data-sharing.
Business Impact: LLMs effectively serve as virtual research assistants, enabling faster decision-making and knowledge discovery across the organization. This is particularly critical in life science and pharmaceutical settings, where timely access to the right information can make or break a project.
Cost Reduction Strategies Through AI/ML
Here are some clear ways AI/ML can cut down drug development costs:
- Reduced Trial Failures: By improving target selection and lead compound discovery, AI dramatically reduces the number of failed candidates in later stages. Every failed candidate can represent tens or hundreds of millions of dollars in wasted investment.
- Smaller, More Targeted Trials: Adaptive trial designs and predictive analytics allow for smaller, more strategically designed trials, lowering recruitment and operational costs.
- Operational Efficiency: Automation and robotics, guided by AI, cut down the manual labor needed for repetitive tasks in labs and clinical settings.
- Data Management: Cloud-based AI platforms help manage massive datasets efficiently, reducing infrastructure costs and providing scalability.
- Regulatory Streamlining: Streamlined submission processes and proactive safety monitoring reduce compliance issues and the risk of expensive delays.
Collectively, these factors can shorten the standard drug discovery timeline, often by months or even years, leading to earlier market entry and extended patent life—both of which are substantial drivers of ROI.
Key Considerations and Best Practices
For CEOs, CTOs, CFOs, Directors of Operations, and VPs of Operations in pharmaceutical and life science organizations looking to implement AI/ML in drug discovery, here are important considerations:
- Data Quality and Integration: AI/ML algorithms thrive on high-quality, well-integrated datasets. Invest in data cleaning and governance to ensure your models are fed robust, reliable data.
- Skilled Talent and Partnerships: Building AI solutions requires cross-functional expertise. Consider hiring or partnering with bioinformaticians, data scientists, chemoinformaticians, and IT consulting teams experienced in life science workflows.
- Scalable Infrastructure: AI workloads can be compute-intensive. Evaluate cloud and edge computing options for scalable processing power, and ensure your IT infrastructure can handle massive data volumes.
- Regulatory Strategy: Work closely with regulatory agencies and maintain thorough documentation. Demonstrate model validity and traceability to ensure compliance.
- Security and Privacy: Handling sensitive clinical and patient data necessitates robust cybersecurity measures. Compliance with HIPAA, GDPR, and other data regulations is paramount.
- Pilot Programs: Start with pilot projects that tackle low-hanging fruit, such as compound screening or patient recruitment. Once the ROI is clear, scale up gradually.
- Change Management: Implementing AI solutions often requires cultural shifts. Encourage training and stakeholder engagement to ensure teams are aligned and ready to embrace new tools.
The Future Outlook
We are in an era where AI/ML and LLMs are not just add-ons but essential drivers of innovation in drug discovery. Looking ahead:
- Precision Medicine Evolution: As personalized therapies become mainstream, AI will help design drugs tailored to individual genetic profiles, drastically enhancing treatment efficacy.
- Integration with Gene Editing: Tools like CRISPR will leverage machine learning to identify off-target effects more accurately, making gene therapy safer.
- Real-Time Regulatory Oversight: Regulatory bodies are increasingly aware of AI’s potential and may begin adopting real-time data monitoring for faster, more dynamic approvals.
- Fully Automated Labs: The concept of “Lab of the Future” will feature end-to-end automation, from compound design to in vivo testing, all orchestrated by AI solutions.
Companies investing in AI and LLMs today will be the ones shaping the future of pharmaceutical breakthroughs. This transformative technology is no longer a luxury—it’s becoming a competitive necessity.
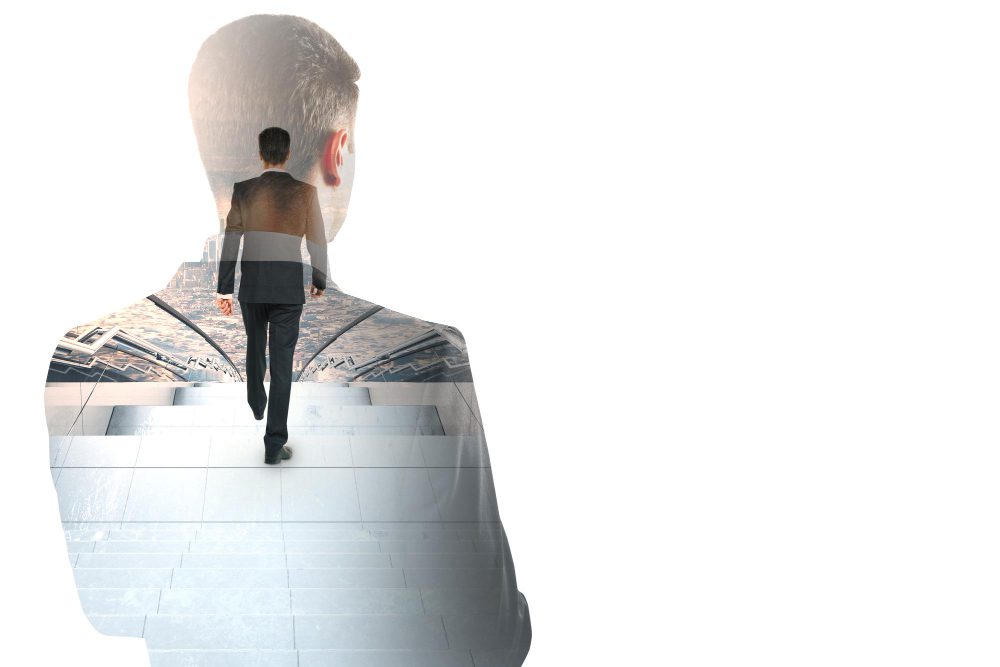
Bring your clinical idea and let us help you to make it reality
Conclusion
AI has fundamentally altered the drug discovery landscape by speeding up the drug development pipeline, cutting costs, and improving success rates. From target identification to lead optimization, clinical trial management, and regulatory compliance, AI/ML solutions can address the most pressing challenges faced by life science and pharmaceutical organizations.
For CEOs, CTOs, CFOs, Directors of Operations, and VPs of Operations, the message is clear: AI and LLMs are key levers for operational efficiency and market leadership. By adopting a well-planned approach to data governance, infrastructure, talent acquisition, and change management, you can unlock the full potential of these technologies.
Embracing AI/ML today isn’t just about staying competitive—it’s about shaping the future of drug discovery. With the right strategy, investment, and collaboration, life science organizations can dramatically accelerate their R&D timelines, bring life-saving treatments to patients faster, and strengthen their bottom line in a rapidly evolving industry.
Key Takeaways
- AI/ML and LLMs offer substantial time and cost benefits in drug development, from initial target identification to clinical trials and post-market surveillance.
- High costs and long timelines in pharmaceutical R&D can be mitigated by predictive modeling, virtual screening, automated workflows, and data-driven decision-making.
- The future of drug discovery will increasingly revolve around personalized medicine, real-time data monitoring, and fully automated labs driven by AI.
By integrating these technologies effectively, the life science and pharmaceutical sector can look forward to a faster, more efficient, and cost-effective route to transformative medical breakthroughs.
Discover more from WinFully on Technologies
Subscribe to get the latest posts sent to your email.